TimeCLR: A Contrastive Learning Based Framework for Video Classification
using new deep architectures to learn the state evolution of a variety of chaotic dynamical systems and significantly extend the prediction time.
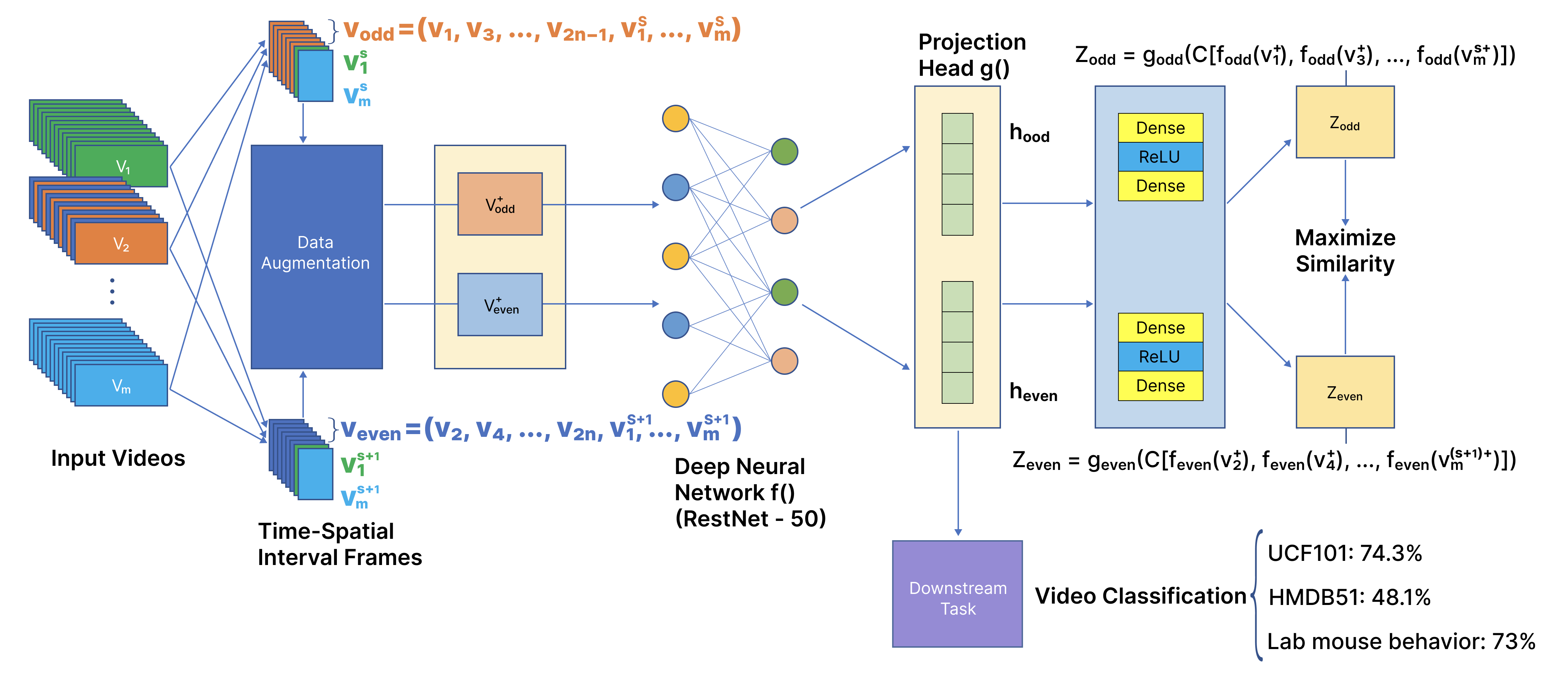
Abstraction
We propose a new contractive learning-based framework, named TimeCLR, for video classification which can capture the global context in the video by our proposing Spatial-Temporal Segment Frame Selection and enhance the inherent sequence structure of the video by our proposing Spatial-Temporal Interval Frame Selection. The goal of contrastive learning is to learn an encoder that encodes similar data as close as possible and makes the encoding results of different data as far away as possible. Compared with generative learning, contractive learning does not need to pay attention to the cumbersome details of the instance, but only needs to learn to distinguish the data in the feature space of the abstract semantic level, so the model and its optimization become simpler. Fundamentally speaking, contrastive learning has a stronger generalization ability. In addition, its other advantage is that it allows us to flexibly define powerful loss targets, as long as we can find a reasonable way to make positive and negative samples for comparison. However, in the current video field, existing methods rely to a large extent on short-range Spatiotemporal meanings to form cut-level contrast signals, which limits their use of global context. Therefore, we proposed TimeCLR to solve this problem. A large number of experiments have shown that our video-level contrastive learning framework (TimeCLR) is superior to the most popular model in video classification.
Citation
@inproceedings{,
title = {TimeCLR: A Contrastive Learning Based Framework for Video Classification},
author = {Chengjie Zheng, Shiqian Shen, Wei Ding, Ping Chen},
year = {2021}
}